Product News
June 23, 2020
The ‘Digital Twin’ in Hardware in the Loop (HiL) Simulation: A Conceptual Primer
Michael Grieves first uttered the term ‘digital twin’ in 2003, and since then much ink and many pixels have been spilled over it. We’ll keep it relatively brief. While ‘digital twin’ can mean many things to many people, it has a more restricted set of understandings when we speak in the context of real-time simulation and/or Hardware in the Loop (HiL) testing.
To begin to explain this closer-cropped meaning, let’s start by thinking of a mirrored copy of a hard drive. It’s a periodically-updated copy of a hard drive in current use, intended to be redundant if/when required. The contents of the mirrored drive can be validated as a duplicate of the source drive at any given time. So it’s a four-dimensional protective mechanism (length/width of data, bit depth, plus the dimension of duration, or time) of a hard drive. Yet already this metaphor lacks the complexity required to outline everything we need to speak about here adequately.
The Iron Bird in the Digital Era
An additional metaphor may serve us better here. In the aircraft design and engineering cycle, which includes MEA (More Electrical Aircraft), a concept exists known as the ‘iron bird’—an integration test rig. All the systems and subsystems of an aircraft are assembled, laid out on the floor of a hangar, say, so that the entire plane is, in essence, operational, except the chassis itself–but it is not physically in the air.
Now to clarify: the iron bird, at the time of its inception in circa 1985, was a hybrid physical/simulated plane during a time where planes evolved exponentially more numerous and more complex systems. (Reasons for more in-air tests as these ‘systems of systems’ grew not being more common should be obvious: massive expense, countless permutations and combinations, huge development times, loss of life, etc.)
All the constituent pieces of this assemblage are validated, and are receiving live stimuli and issuing reactions and outputs as though the plane itself were flying–but interactions with engines, landing apparatus, wing flaps, etc. may or may not be virtual, depending on the phase of Verification and Validation (V & V). The iron bird testing phase must be as good (validated, accurate, entirely reproducible) as ‘real life’ i.e., as a real-world flight—there is very little to no ‘wiggle room’ in aviation–or this testing phase and concept serves no purpose.
The use of iron birds for aerospace V&V, where physical components are partly replaced with digital/virtual parts and through using real-time simulation—again, depending on the V&V phase—allows aircraft manufacturers and their equipment developers to save vast fortunes of money on expensive prototyping. Though of course, at the end of the day, virtual models need some more extensively validated dynamics and response validation before they are ready to jet you off to your next sunspot vacation.
A Digital Twin: A Great Deal More Than Just a Simulator
Now, if we add some other elements to the duplication/redundancy notion (see: the mirrored copy of the hard drive), and add some levels of complexity, interchangeability, and communications (see: the iron bird), the functioning of what we currently mean by ‘digital twin’ in the context of real-time simulation (or HIL) begins to be clearer.
If we were to break it down functionally by what it does, is able to do, and at what it conceptually excels in our current reading:
- The digital twin can read data to/from its physically operating counterpart and report on itself via its hardware/software surroundings to an overseeing entity—for maintenance, for logging, for reporting, for control, etc.
- Meaning: the digital twin—itself virtual–has a bridge of connectivity with the ‘real-world’
- The digital twin has a profile of its own in the form of a dynamic model and related parameters, and it is adjusting itself, in close comparison, with its physical counterpart in real-time or ‘near real-time’
- Thus, it is self-adaptive
- The digital twin is able to describe its inner components’ interactions in much greater detail than its physical counterpart can, since the latter has a limited amount of sensoring
- Thus, it can act as a one-step removed, in-depth observer
- The digital twin can respond to the same stimuli as its physical counterpart and help identify abnormal operations, malfunctions and identify the source of the problem
- Thus, it is a situationally-aware and decision-supporting tool
- The digital twin can respond to synthetic stimuli and help in obtaining what-if scenario analysis that cannot be analysed at will through its physical counterpart due to practical concerns. In this way, it will differ from a simulation (or real-time simulation) as it is a validated dynamic replica of its physical counterpart
- Thus, it is extremely powerful as a predictive tool, and can be used additionally as a design and planning tool
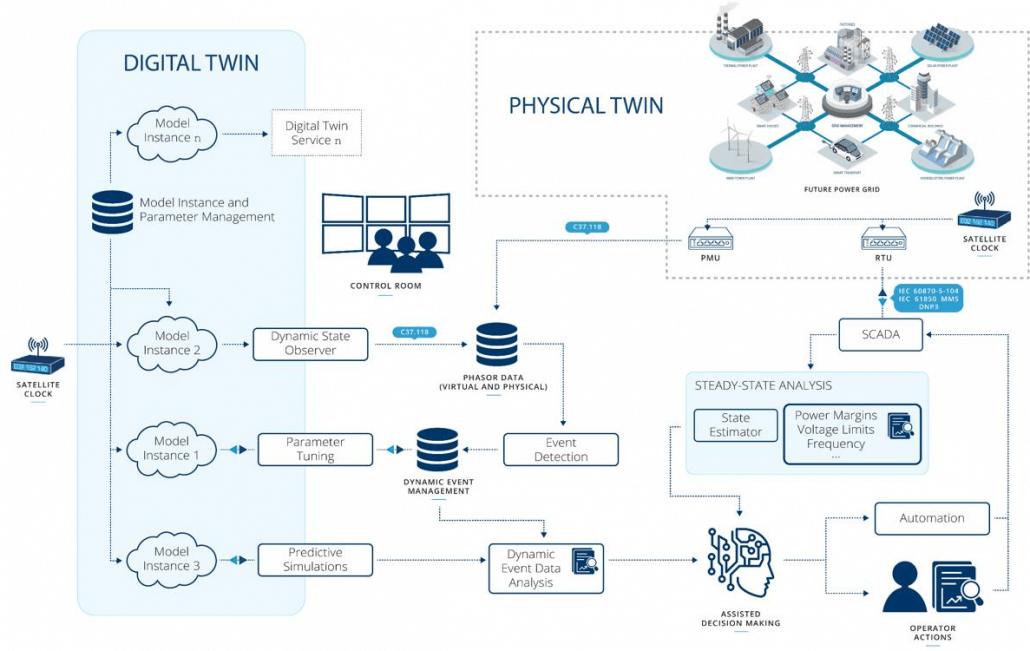
Digital Twins: The Current State of Affairs
As a real-time simulation afficionado, the potential uses should be becoming clear to you by this point, but to give some genericized examples of current uses:
- An electrical car manufacturer can accumulate vast volumes of data on its vehicles during their operation. The data is pivot-table ready to the extent that it can be logged, viewed, and analyzed by driver type profile, geographical location of car, and many other slants or viewpoints on the data that may unearth valuable and/or useful results. (One of ‘big data’s’ chief strengths is the myriad of angles from which it can be read, each new angle potentially offering new insight.) Through this, the manufacturer can send notifications to the local garage, or to the end user, when a Battery Management System has unexpected behaviour, for example, or for engine part replacement.
- An electric utility can learn about usage/consumption patterns through this combination of logging/reporting, AI, and vast amounts of data to automate what is now called Demand Response, through adjusting residential boilers and heaters to ensure sufficient spinning reserve or stable operation.
- In the near future, predictive maintenance where—before failure has even occurred in a consumer good—a potential future breakdown can be predicted, all the possible routes towards a positive outcome can be analyzed, and action can be taken, before the user is even aware anything untoward has happened.
Into the Great, Wide-Open Future
We’re on the cusp of this particular combination of present and future-looking enhancements that can be applied across many categories for the benefit of design, prototyping, regular use and maintenance/replacement. The clustering of various recent phenomena has made this approach and all of its implicated uses possible: big data, AI, 5G and faster networks, cloud computing, and the Internet of Things, among others.
How will ‘digital twins’ help in the next 50 years and onwards for power grid operation? They’ll help stabilize our power systems through diagnosis, monitoring, experience, and predict through ‘what if’ scenarios. They are, in a way, digital time travel: going back in time to examine histories of aggregated data; going forward to predict outcomes. They’ll help us learn to operate our power grids better and more safely; and they’ll provide many times more and better test coverage for upgrades and improvements.
As they do all of this quietly and reliably, they’ll also provide better, more numerous, and more detailed data sets, and thus improve the training and output of AI immeasurably. They’ll also allow us to explore edge/corner cases we might never have thought of even simulating, based on their physical twin’s data. They will help in finding countermeasures to prevent wide area failures and to decrease grid downtime. They’ll improve autonomous system operation, and they’ll help optimize our simulation scenario selection through model-trained AI.
‘Digital twin’ is a concept where the language has been used fairly loosely thus far–yet combinations of the advances made possible through this thinking promise exciting and inevitable advances for real-time simulation. It’s not a matter of if this concept and its associated wholesale improvements and nuances are leveraged, but when and how.
Learn more
Watch the recorded RT20 Panel Discussion on Digital Twin to Increase Resilience of Power Grid